Artificial Intelligence in Boosting Semiconductor R&D
The semiconductor industry has witnessed tremendous growth in recent years, fueled by advancements in technology and increasing demand for more powerful, efficient, and smaller devices.
As the industry progresses, research and development (R&D) professionals face growing challenges in improving device performance and reducing manufacturing costs. Artificial intelligence (AI) has emerged as a powerful tool to help overcome these challenges by accelerating the R&D process and offering innovative solutions.
In this article, we’ll explore the various ways AI is being employed in semiconductor R&D, from materials discovery to manufacturing optimization, and how it is shaping the future of the industry.
AI-Powered Materials Discovery
One of the most significant advancements in semiconductor R&D is the use of AI in discovering new materials. Machine learning (ML) algorithms can analyze vast amounts of data and predict properties of materials, accelerating the process of identifying candidates for next-generation devices. For instance, AI can be utilized to search for new high-performance materials with specific properties, such as higher conductivity or increased thermal stability.
Researchers at MIT have developed an ML-based technique that can rapidly evaluate new materials, reducing the time and resources required for experimentation. By incorporating AI into the materials discovery process, R&D professionals can accelerate innovation and develop new semiconductors with enhanced performance.
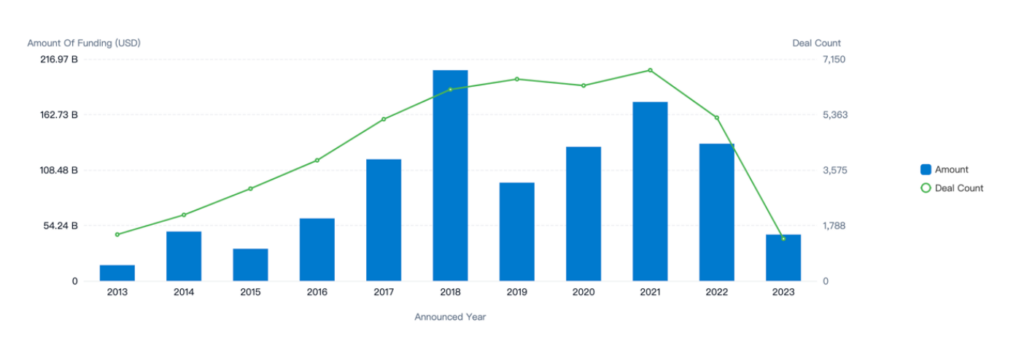
Since 2013, there has been a steady increase in funding for artificial intelligence and semiconductors, reaching a peak in 2018 with 6,170 deals totaling $206.12 billion. However, investment in the industry has seen only slight increases since 2019, and in 2021, funding levels have not returned to previous highs. One possible explanation for this trend is the ongoing shortage of semiconductors, which remains a significant challenge for many companies. The uncertainty around when this shortage will be resolved may be causing some investors to pull back from the industry temporarily.
AI-Driven Design and Simulation
Semiconductor design and simulation are complex processes that require significant computational resources. AI can be leveraged to optimize these processes, resulting in faster design cycles and improved device performance. One such application is in electronic design automation (EDA), where ML algorithms can predict optimal circuit layouts and component placement.
AI can also enhance the accuracy of simulations by learning from previous iterations and incorporating this knowledge into future simulations. For example, a research group at Stanford University has developed an AI-driven simulation tool that can model the behavior of transistors with a high degree of accuracy. This approach allows R&D professionals to rapidly iterate and refine their designs, ultimately leading to more efficient and reliable semiconductors.
AI-Optimized Manufacturing Processes
Semiconductor manufacturing is an intricate and highly controlled process. AI has the potential to significantly improve manufacturing efficiency and yield by identifying potential issues and optimizing various production parameters. ML algorithms can be employed to analyze large volumes of data from the manufacturing process, identifying patterns and correlations that would be difficult for humans to detect.
For example, AI can be used to optimize lithography, a critical step in semiconductor manufacturing that involves the precise transfer of circuit patterns onto silicon wafers. Researchers at the University of California, Berkeley, have developed an AI-based approach that can optimize lithography parameters, resulting in improved pattern fidelity and reduced defects. This application of AI not only streamlines the manufacturing process but also helps in reducing the overall production costs.
Additionally, AI-driven predictive maintenance can minimize equipment downtime and improve overall efficiency in semiconductor fabrication plants. By analyzing data from sensors and equipment logs, ML algorithms can predict potential failures and suggest preventive measures, ensuring smooth operations and reducing the risk of costly disruptions.
AI-Enhanced Quality Control and Defect Detection
Quality control is a crucial aspect of semiconductor manufacturing, as even minor defects can lead to device failures and reduced performance. AI can be used to improve the accuracy and efficiency of defect detection, by employing ML algorithms to analyze images captured during the inspection process. These algorithms can learn to identify defects, such as cracks or particle contamination, with high precision, enabling faster and more reliable quality control.
A research team at the University of Illinois has developed a deep learning-based system for automated defect detection in semiconductor wafer images, demonstrating improved accuracy and reduced false positives compared to traditional inspection methods. By incorporating AI into quality control processes, R&D professionals can ensure the production of high-quality, reliable semiconductors.
Closing Thoughts:
Artificial intelligence is playing an increasingly significant role in semiconductor research and development, as it accelerates innovation, enhances performance, and reduces manufacturing costs. By leveraging AI in materials discovery, design and simulation, manufacturing optimization, and quality control, R&D professionals can develop next-generation semiconductors that meet the growing demands of the industry.
As AI continues to advance, its applications in the semiconductor sector are expected to expand further, driving new discoveries and pushing the boundaries of what is possible. The integration of AI in semiconductor R&D not only helps the industry tackle current challenges but also paves the way for a more innovative, efficient, and sustainable future.